Goal: The objective of this current project is to develop and validate different modelling approaches, such as non linear and non-parametric regressions, artificial neural networks and Genetic Algorithms to develop more accurate models with the ultimate goal to make more precise predictions of weed seedling emergence to provide growers with consistent tools to make better weed management decisions
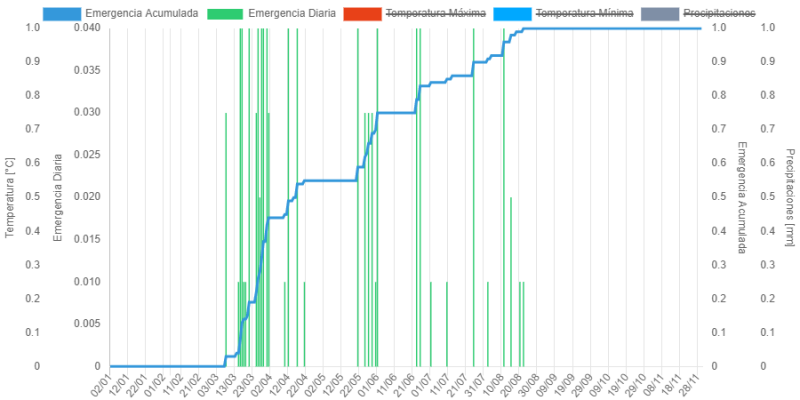